The Evolution of Artificial Intelligence: A Comprehensive Overview
The current landscape of AI brims with exciting possibilities, marked by a synergy of technological advancements and innovative research endeavors. From the depths of machine learning to the horizons of neuromorphic computing, AI's trajectory continues to
2024-08-01 11:28:00
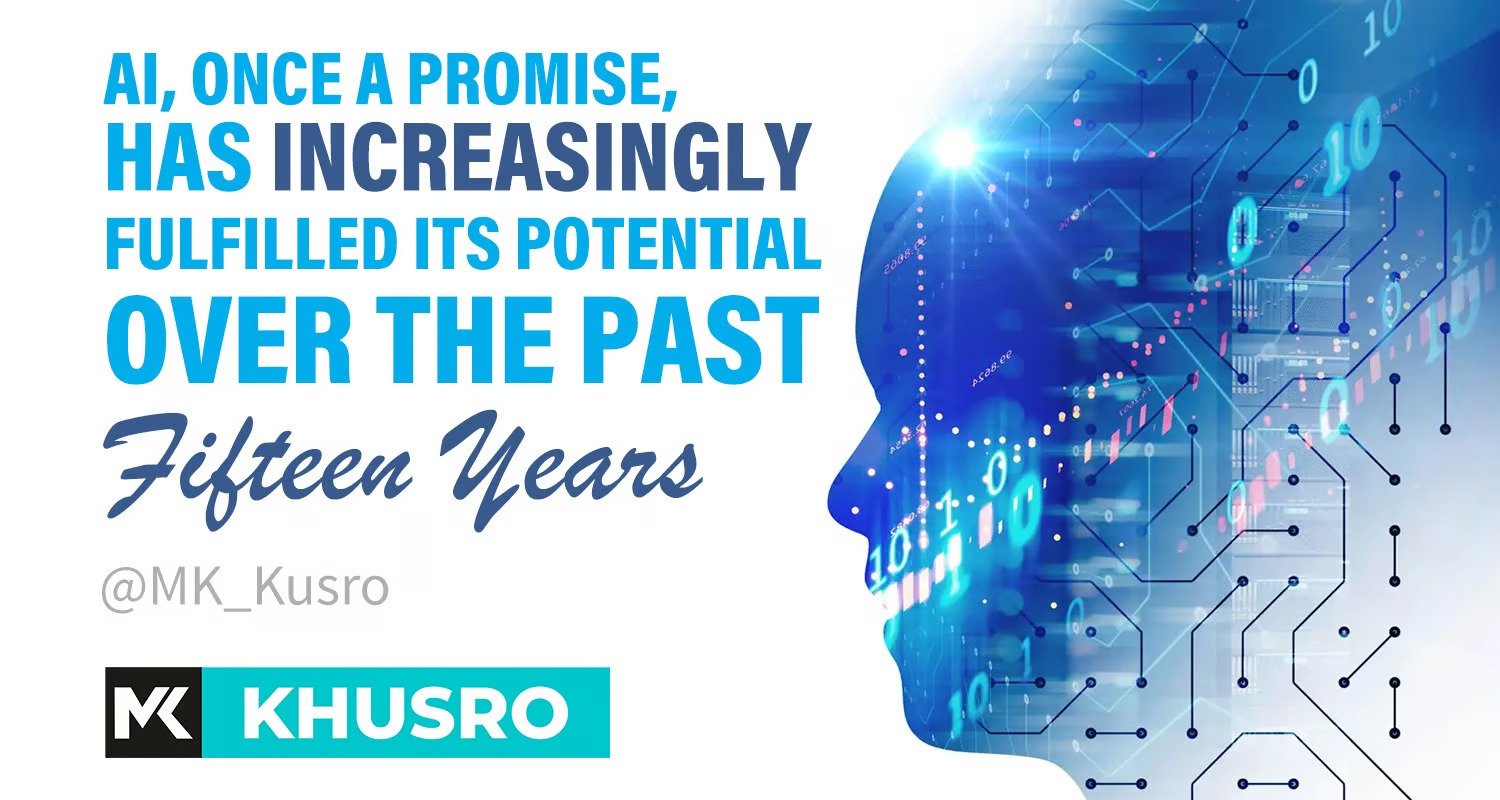
The landscape of Artificial Intelligence (AI) has undergone a radical transformation in recent years, reshaping the way we perceive, interact, and benefit from technology. Until the brink of the millennium, AI was largely steeped in its potential, a realm of promises yet to be fulfilled. However, the last fifteen years have seen a profound redemption of those promises, with AI now permeating various facets of our lives. As it continues to ascend as a pivotal force in society, the focus has shifted from merely crafting intelligent systems to creating intelligent systems that are not only human-aware but also trustworthy.
Factors Fueling the AI Revolution
Several key factors have fueled this monumental AI revolution. Foremost among them is the maturation of machine learning, buoyed by the availability of cloud computing resources and the widespread collection of data via the web. Machine learning, propelled significantly by "deep learning," a subset of adaptive artificial neural networks trained through backpropagation, has marked a leap in information processing algorithms' performance. This surge in algorithmic efficiency coincides with substantial advancements in hardware technology for fundamental operations such as sensing, perception, and object recognition. Additionally, the emergence of new platforms and markets for data-driven products, coupled with economic incentives to explore novel territories, has substantially contributed to the advent of AI-driven technology.
1. Large-scale Machine Learning
The bedrock problems in machine learning, including supervised and unsupervised learning, are well-established. Current efforts predominantly revolve around scaling existing algorithms to accommodate vast datasets. Unlike traditional methods that iterated over data multiple times, modern algorithms strive to process data in a single pass, with some instances relying on sublinear methods, examining only a fraction of the dataset.
2. Deep Learning
The triumph in training convolutional neural networks has revolutionized computer vision, catalyzing applications like object recognition, video labeling, and activity recognition. Deep learning's impact extends to other domains of perception such as audio analysis, speech recognition, and natural language processing, pushing the boundaries of what machines can perceive and comprehend.
3. Reinforcement Learning
Unlike traditional machine learning, reinforcement learning pivots towards decision-making. This technology facilitates AI's deeper integration into real-world learning and action execution. Despite its decades-long existence, reinforcement learning faced challenges regarding representation and scaling until the infusion of deep learning breathed new life into it. Notably, AlphaGo's triumph over the human Go champion stemmed largely from reinforcement learning, showcasing its potential in action.
4. Robotics
While static robotic navigation puzzles are mostly resolved, contemporary efforts focus on training robots to interact adaptively with their surroundings. Manipulation within interactive environments emerges as a pivotal area of interest. The integration of deep learning into robotics remains nascent due to the complexity of acquiring substantial labeled datasets. Reinforcement learning offers promise but necessitates safe exploration of policies without adverse consequences.
5. Computer Vision
Deep learning's ascendancy has reshaped computer vision, shifting from conventional support vector machines to neural networks. The amalgamation of ample computing power, especially GPUs, vast datasets available on the internet, and neural network refinements has elevated computers' visual classification prowess, even outperforming humans in certain tasks. Ongoing research focuses on automating image and video captioning to further enhance AI's visual capabilities.
6. Natural Language Processing
Coupled with automatic speech recognition, Natural Language Processing (NLP) stands as a vibrant domain within machine perception. It is swiftly becoming ubiquitous for mainstream languages boasting substantial datasets. The recent achievement of real-time translation and Google's revelation that a significant portion of mobile queries transpires through voice highlight NLP's rapid evolution. Current research gravitates towards developing sophisticated systems capable of interactive dialogues, surpassing mere response to structured requests.
7. Collaborative Systems
The realm of collaborative systems delves into models and algorithms facilitating autonomous systems' collaboration with other systems and humans. This research hinges on formal collaboration models and studies the requisite capabilities for effective partnership development. The emphasis lies in leveraging human-AI synergy to transcend limitations and augment activities mutually.
8. Crowdsourcing and Human Computation
Recognizing the superiority of human intelligence in numerous tasks, crowdsourcing and human computation explore methods to enhance computer systems by integrating human intellect. These domains, established around fifteen years ago, have cemented their presence in AI research. Platforms like Wikipedia and crowdsourcing avenues such as Amazon Mechanical Turk exemplify harnessing human intelligence innovatively. Current endeavors aim to delineate optimal task divisions between humans and machines, capitalizing on their distinct capabilities and costs.
9. Algorithmic Game Theory and Computational Social Choice
AI's purview extends to economic and social computing dimensions, encompassing incentive structures and addressing potentially misaligned incentives. Distributed AI, multi-agent systems, and computational mechanism design have garnered attention, navigating the landscape of self-interested human participants or AI-based agents representing them. Research areas include computational social choice, incentive-aligned information elicitation, and algorithmic game theory, aiming to foster incentive-compatible systems and address various incentive-related challenges.
10. Internet of Things (IoT)
The concept of interconnecting an extensive array of devices to collect and share sensory information is the focal point of IoT research. While technology and wireless networking facilitate device connectivity, AI plays a pivotal role in processing copious amounts of resultant data for intelligent purposes. The challenge lies in harmonizing the multitude of incompatible communication protocols across these devices, an area where AI intervention could prove instrumental.
11. Neuromorphic Computing
Departing from the traditional von Neumann model of computing, neuromorphic computing models draw inspiration from biological neural networks. Manufacturers are actively pursuing alternative computing models to enhance hardware efficiency and robustness. Although neuromorphic computers are still in their infancy, their potential to complement conventional von Neumann architectures, especially in executing deep neural networks, holds promise for the future landscape of AI applications.
Hot Areas of Research in AI
Conclusion
The current landscape of AI brims with exciting possibilities, marked by a synergy of technological advancements and innovative research endeavors. From the depths of machine learning to the horizons of neuromorphic computing, AI's trajectory continues to evolve, propelling us into an era where intelligent systems not only perceive but also comprehend, interact, and collaborate, promising a transformative future rich with possibilities and potential.
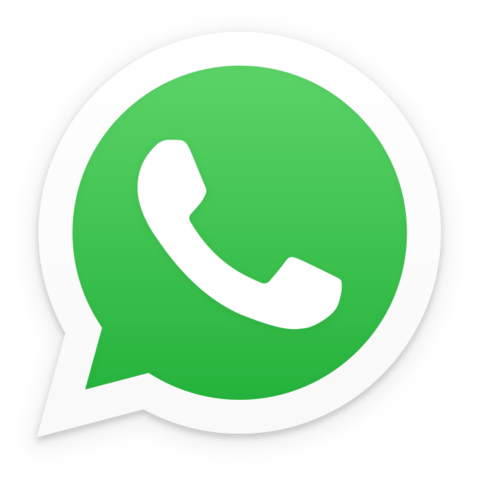
© 2024 MK Khusro. All rights reserved.